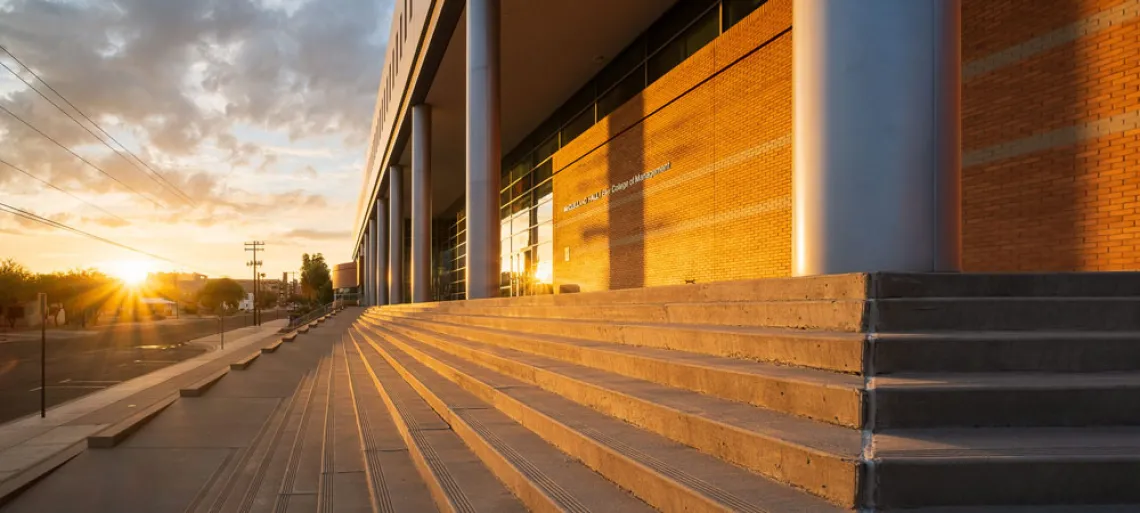
When
Where
PhD Candidate, Naveen Jindal School of Management, University of Texas at Dallas
Pragmatism-Aware Knowledge-Data Fusion: Integrating External Domain Insights with Internal Data
Abstract: The boom of machine learning and AI in the last two decades has enabled various business organizations to effectively utilize their extensive internal data. However, they often struggle with leveraging external general knowledge that varies in modality, granularity, and generality. Such external general knowledge is prevalent, existing in forms such as professional industry reports and discussion threads on online forums. This external knowledge can provide in-depth insights beyond internal data, yet integrating it with internal transaction data for specific business tasks remains unclear. To address this, this study introduces a novel knowledge-data fusion framework comprising two key components: knowledge representation and knowledge incorporation. For knowledge representation, we explored three distinct forms of external knowledge: raw knowledge, latent knowledge, and relational knowledge. For knowledge incorporation, we developed a “pragmatism-aware (PA) distillation” model by including an intermediate pragmatic teacher in the knowledge distillation process to bridge the gap between the conventional teacher model and the student model. This PA distillation model captures the principles of external general knowledge while aligning with the nuances of internal data. We demonstrated the efficacy of the PA distillation model for the specific task of content creation and sales prediction for non-fungible tokens (NFTs), combining internal NFT transaction data with external knowledge from Reddit discussions. Our model was compared with several state-of-the-art knowledge incorporation approaches, including data fusion and knowledge distillation. The results show that the PA distillation model significantly enhanced the prediction of NFT sales, achieving a 22.06% increase in F1 score compared to the baseline model using only NFT transaction data. Furthermore, it outperformed the data fusion model by 4.99% and the knowledge distillation model by 4.82% in F1 scores. Additionally, our model also facilitates better NFT creation and monetization: incorporating valuable external knowledge directly into NFT creation processes through generative AI models resulted in a 2.1% rise in sale probabilities. This paper lays the design foundation for future research on knowledge-data fusion and offers significant practical implications for utilizing external general knowledge.
Bio: Hong Zhang is a Ph.D. candidate in Information Systems at the University of Texas at Dallas, specializing in the intersection of Fintech platforms and AI. Her dissertation focuses on key issues in two-sided Fintech platforms, including market growth strategies, trading efficiency, and integrating external knowledge with internal transaction data in AI models to enhance decision-making outcomes. She extensively develops and applies methodologies such as machine learning, econometrics, and deep learning in her research. Currently, Hong’s work is under advanced revision in Management Science and MIS Quarterly, and she has presented her research at various leading IS conferences such as CIST, ICIS, and WITS. Her dissertation was recognized as a Best Dissertation Award finalist at WITS 2024, and her research has received multiple best paper runner-up or finalist awards at CIST, WITS, and the Boston Platform Strategy Symposium. In 2024, she was honored as the UT Dallas JSOM Ph.D. Student of the Year.