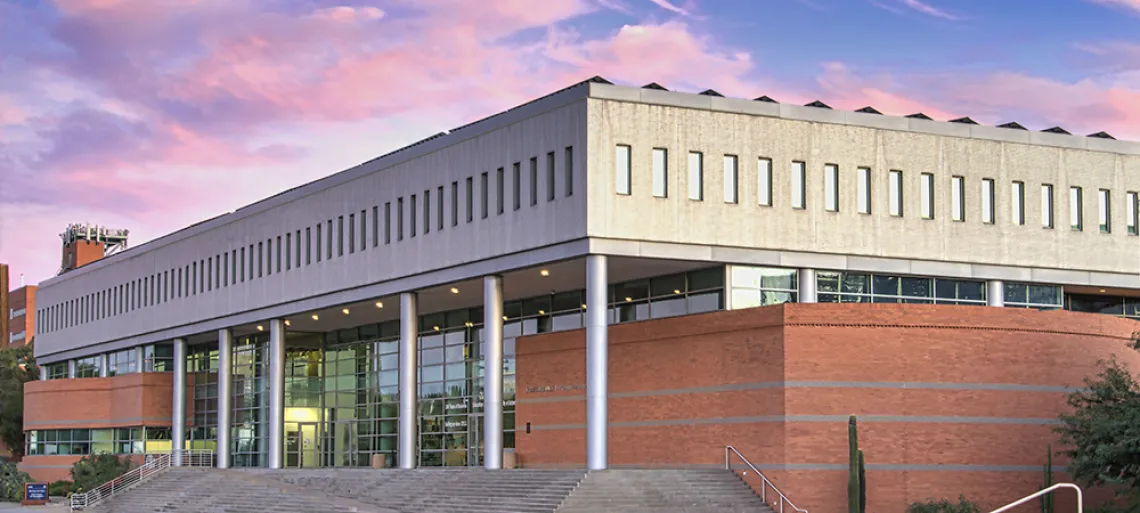
When
Where
Professor, University of Wisconsin Milwaukee
Generative AI as an Information Intermediary: A Novel Deep Learning Method for Financial Risk Prediction
Abstract: With integrated development of financial markets and information technology, non-financial information, especially information carried in disclosure reports, plays an increasingly important role in conveying financial risk signals. Such signals are embedded in not only the surface meaning but also, more significantly, the latent meaning of a report. While previous studies on financial risk prediction incorporating disclosure reports have mostly focused on capturing the surface meaning, the latent meaning is still largely untapped, and the core surface meaning remains elusive. To unleash the full incremental predictive value of disclosure reports, we initiate a new avenue, generative AI-enhanced financial risk prediction. We position generative AI as an information intermediary and propose a functional analogy framework to conceptualize the process of leveraging disclosure reports with four functions: perception, extraction, reasoning, and evaluation. We then propose a novel deep learning method to implement these functions. We design a function-based bidirectional representation module to explicitly and separately extract representations for the emphasis information produced by the extraction function and insight information produced by the reasoning function, guided by tailored convergent and divergent mutual information criteria, respectively. We also design a hard-and-soft transformer module to capture embedding-level correlations both within and between financial and non-financial information. Empirical evaluation at the model level (in terms of discrimination performance and out-of-time prediction performance) and impact analysis at the application level (in terms of identification performance) demonstrate advantages of the proposed method over benchmarked state-of-the-art methods on all fronts. Mechanism-level analyses further reveal the core drivers underlying the proposed method, including the performance-enhancing effect of every design artifact, the ability to identify and leverage emphasis and insight information for generating discriminative representations, and the generalizability across predictive contexts.
Bio: Huimin Zhao is a Professor of Information Technology Management and Roger L. Fitzsimonds Distinguished Scholar at the Lubar College of Business, University of Wisconsin-Milwaukee, USA. He received the Ph.D. degree in Management Information Systems from the University of Arizona, USA. His current research interests include artificial intelligence and data analytics. He has published in such journals as Information Systems Research, MIS Quarterly, Journal of Management Information Systems, Communications of the ACM, ACM Transactions on MIS, IEEE Transactions on Knowledge and Data Engineering, IEEE Transactions on Systems, Man, and Cybernetics, Information Systems, Journal of the AIS, and Decision Support Systems. He currently serves as an associate editor for Information Systems Research and the Journal of Business Analytics, and has served in the past as a senior editor for Decision Support Systems and an associate editor for MIS Quarterly.