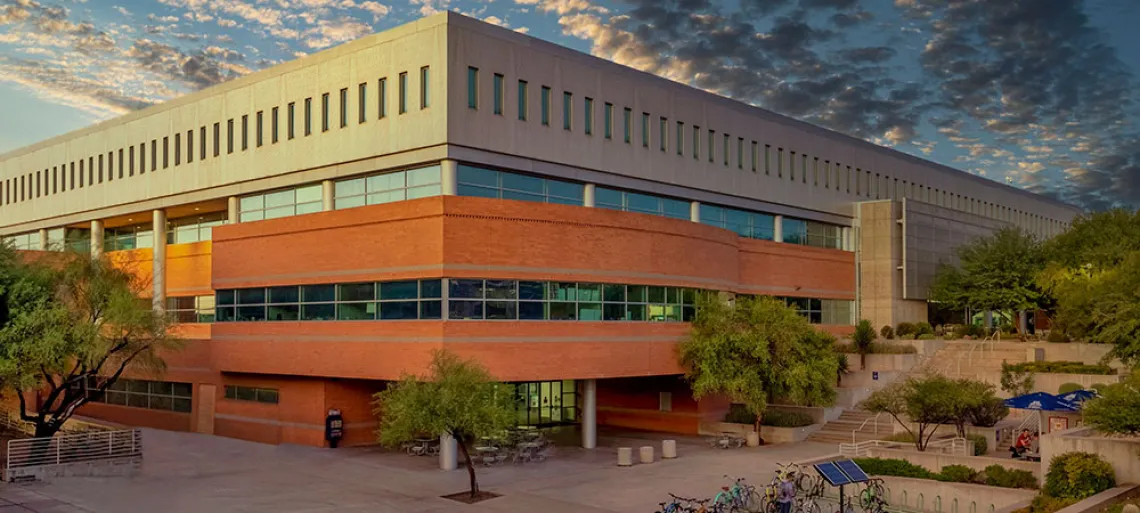
When
Where
Resolving AI Value Paradox: Unlocking AI's Value Through Strategic Knowledge Integration
Abstract: Billions of dollars are being spent on artificial Intelligence (AI) with the promise of radical transformation. Yet, firms struggle to translate such investments and excitement into value. This AI value paradox stems from AI’s nature as a general-purpose technology with versatility and pervasiveness. As such, AI innovation needs to cross industrial boundaries and be combined with complementary assets specific to the firm or the target industry. I conceptualize this AI through a combinatorial search perspective. Firms pursuing AI innovation with local search by combining cross-boundary AI knowledge with their existing knowledge are expected to have a negative impact on their market value, while those pursuing AI innovations with distant search by combining cross-boundary AI knowledge with knowledge beyond their knowledge are expected to have a positive impact on market value. I test hypotheses with a longitudinal panel dataset comprising 354,650 patents related to autonomous vehicles, 167,164 and 65,967 scientific papers on AI from the computer science field and mechanical engineering fields, respectively, and accounting information for 1,761 firms, in the automotive and adjacent industries from 2009 to 2020. I use a large language model with one-shot prompting to extract AI keywords from academic papers. Next, I identify AI keywords originating from computer science field that are later used in mechanical engineering field to measure cross-boundary AI knowledge. I then conduct a staggered difference-in- differences analysis to examine whether combining cross-boundary AI knowledge in their innovation generation influences firms' value, how different search strategies lead to varying effects, and how these effects change over time. The results confirm my theoretical predictions that firms’ search strategy to integrate cross- boundary AI knowledge significantly impacts their market value. Specifically, firms taking a distant search strategy with cross-boundary AI knowledge tend to experience higher market returns, while those pursuing a local search strategy face greater challenges. This study contributes to the growing body of AI strategy literature by showing conditions under which firms can address AI value paradox.
Bio: Kaige Gao is a PhD candidate in the Department of Design & Innovation at Case Western Reserve University. Her research interest is the evolution of large-scale dynamic heterogeneous digital innovation. Specifically, in her thesis, she uses advanced computational tools, including dynamic multi-level networks, Graphic Neural Networks, and Large Language Models, as well as econometric tools to understand the evolution of AI innovations. She presented her papers at the International Conference of Information Systems and the Academy of Management. Her papers are under review at leading journals such as the Information Systems Research and Strategic Management Journal. Her teaching interests are advanced data analytics, artificial intelligence, and network modeling. Her thesis committee includes Youngjin Yoo (Chair), Kalle Lyytinen, Aaron Schecter (University of Georgia), and Yoonseock Son (University of Notre Dame).