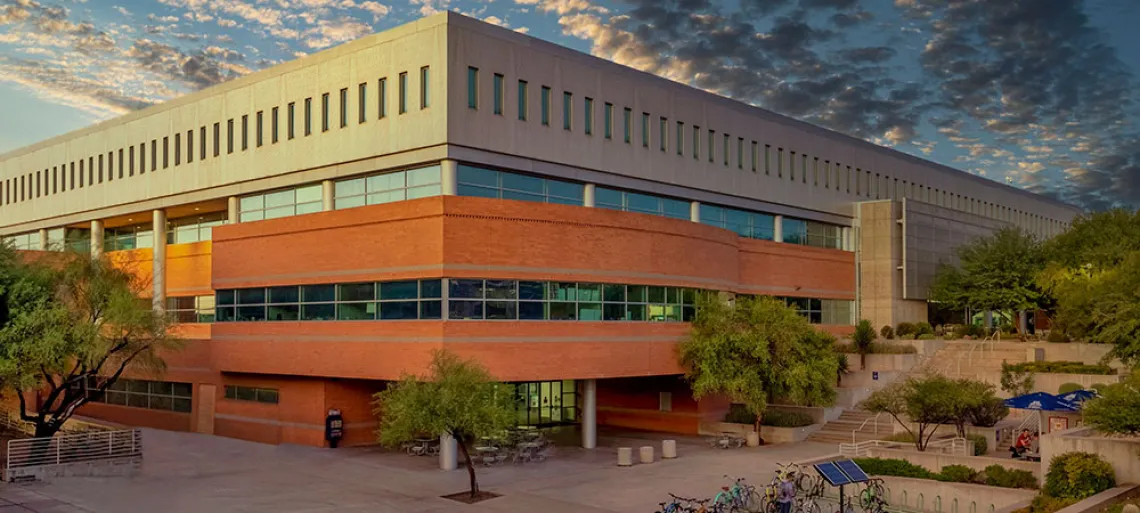
When
Where
Assistant Professor of Information Systems, Foster School of Business, University of Washington
Response-Adaptive Designs in Clinical Trials: Bandit Models for Improved Patient Outcomes
Abstract: Multiple myeloma is an incurable cancer of bone marrow plasma cells with a median overall survival of 5 years. With newly approved drugs to treat this disease over the last decade, physicians are afforded more opportunities to tailor treatment to individual patients and thereby improve survival outcomes and quality of life. However, because the optimal sequence of therapy is unknown, selecting a treatment that will result in the most effective outcome for each individual patient is challenging. This work addresses this challenge, considering the problem of designing personalized treatment recommendations for patients with multiple myeloma using a data-driven analytics method. We formulate the treatment recommendation problem as a Bayesian contextual bandit, which sequentially selects treatments based on contextual information about patients and therapies, with the goal of maximizing overall survival outcomes. We develop a multilevel Bayesian linear Thompson sampling to learn patients’ heterogeneous responses to treatment decisions, which allows us to flexibly account for patient and line-of-therapy level heterogeneity even in the absence of a large number of observations. Facing the difficulty of evaluating the performance of the policy with only observational data, we propose a causal offline evaluation approach to measure the effect of the treatment in the presence of unmeasured confounders. We evaluate the performance of our policy on clinical data collected from 803 patients treated at Seattle Cancer Care Alliance. Our policy achieves a 16.14% predicted improvement in progression free survival compared to the current clinical practice and it outperforms other benchmark strategies. Then we recognize that the treatment effect is only seen at some delayed time after the treatment is provided. The importance of considering delays is highlighted by literature in recent years, while most considerations were motivated by reward delays in advertisement and news article recommendations. As such, the delay was assumed either to be fixed, or stochastic but reward-independent. The more challenging setting of reward-dependent delays was not explicitly addressed previously in the bandit literature. More critically, the “delays” in observing the survival response are the same as the rewards. We propose censored-UCB algorithm that achieves near-optimal regret. Our theoretical results and the algorithms’ effectiveness are validated by empirical experiments.
Bio: Yingfei Wang obtained her PhD of Computer Science from Princeton University in 2017, and afterwards joined Foster School of Business, University of Washington as an assistant professor in Information Systems. Her research lies at the intersection of statistics, machine learning and information systems, focusing on decision-making under uncertainty, exploring the ways where efficient information collection influences and improves decision-making strategies, with applications as diverse as healthcare, e-commerce, recommendation systems, consumer behaviors and inventory controls. Her work has been published in Information Systems Research, Management Science, and top CS conferences.