How Can Free Text Help in Our Understanding of Autism?
UA Eller researchers are using natural language processing to extract mental health diagnostic data from thousands of electronic health records
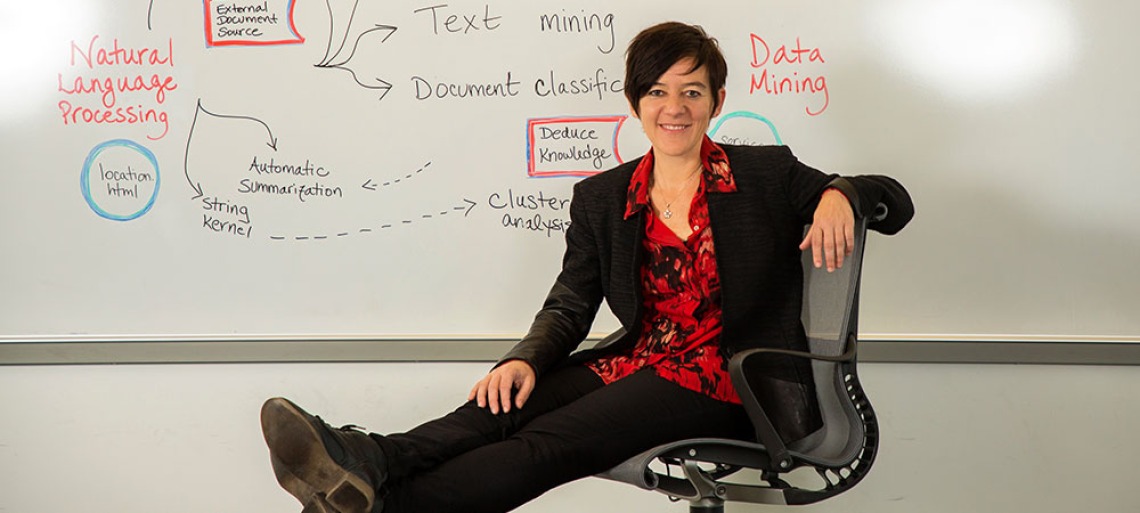
In the not-so-distant future, doctors treating patients with autism spectrum disorder (ASD) may choose treatment plans based on data analyzed from thousands of electronic health records by researchers at the Eller College of Management.
Gondy Leroy, professor of management information systems in the Eller College, is leading a multidisciplinary research team that recently received a grant for $292,404 from the Agency for Healthcare Research and Quality, part of the U.S. Department of Health and Humans Services, to create human interpretable models that automatically annotate the free text in electronic records with the criteria in the Diagnostic and Statistical Manual of Mental Disorders for ASD.
The research team decided to focus on ASD due to the lack of tools to leverage existing records and the unexplained prevalence of the developmental disorder. The records are already collected as part of the Centers for Disease Control and Prevention’s (CDC) ongoing surveillance effort and based on these it is estimated it that 1 in 68 children in the U.S. have autism. The prevalence is 1 in 42 for boys and 1 in 189 for girls. These rates yield a gender ratio of about five boys for every girl. The latest estimate of autism prevalence is up 30 percent from the 1 in 88 rate reported in 2008, and more than double the 1 in 150 rate in 2000.
“Our research focuses on processing records automatically and finding individual diagnostic criteria, while also classifying records as that of a child with or without autism. This will facilitate analyzing millions of records to find changes and trends and to combine with data from other sources,” Leroy said.
Leroy explained that there is a treasure of information already available in electronic health records, but much of the information is in free text and not readily available for large-scale use.
“There are no tools available to extract detailed diagnostic information from the free text in electronic health for large-scale use.” she said.
Noting that these electronic health records are vastly underused, Leroy said the portion ignored most is the free text because it requires advanced natural language processing (NLP) to transform the unstructured information into a structured form for use at a large scale and for integration with other data. A component of artificial intelligence, NLP enables computers to analyze and understand the human language.
Currently, autism surveillance is a manual, costly and slow process that provides basic information about autism cases to the CDC and surveillance investigators. Eller’s research team will address the need for more efficient surveillance techniques for tracking ASD across the country.
“We’ll also address a lack of text processing tools that go beyond discovery of single entities, such as genes or proteins, and provide comprehensive matching to more complex patterns, such as the Diagnostic and Statistical Manual of Mental Disorders criteria. We will design new algorithms that can automatically create these models to annotate and extract complex patterns in text,” Leroy said. “Finally, we will address the need for harvesting large amounts of data available in electronic health records’ free text to supplement existing research projects and to bring new research opportunities through secondary analysis of data.”
The Eller research team will have access to electronic health records for more than 6,000 cases, available through the Arizona Developmental Disabilities Surveillance Program, which contain information on children ages 4-8 in Arizona whose records have been evaluated for presence of ASD. In addition to the original records, a large portion of which is free text, the research team possesses the clinician annotations of records indicating the specific criteria leading to the ASD case assignment (or not).
Leroy’s co-investigators on this two-year project are Mihai Surdeanu, associate professor of computer science at the University of Arizona, Sydney Pettygrove, assistant professor in the UA Zuckerman College of Public Health, and Maureen Kelly Galindo, genetics and developmental research coordinator in the UA College of Medicine.