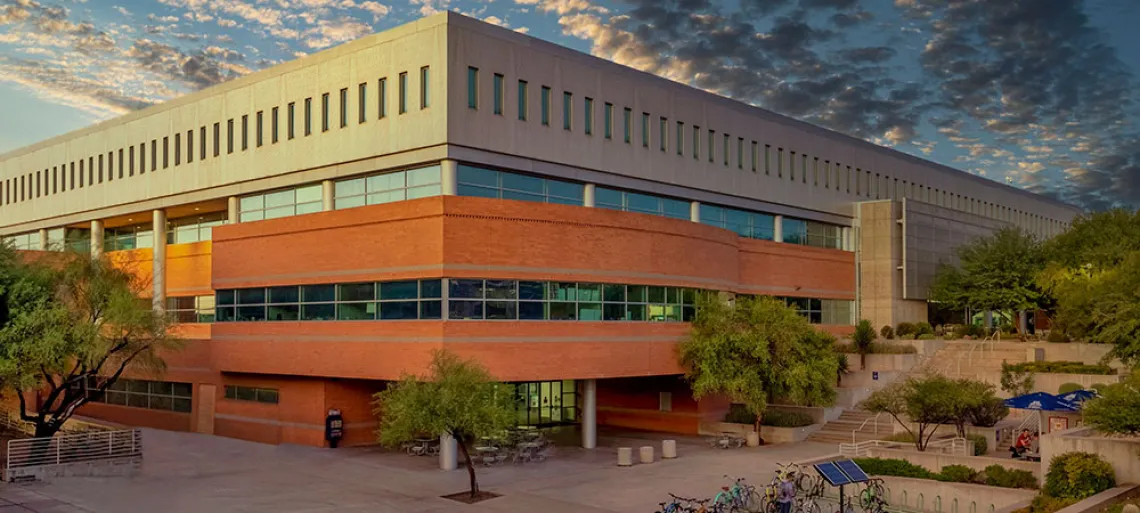
When
Where
Assistant Professor, Department of Computer Science and Engineering, University of Connecticut
Towards Continual Learning on Graphs
Abstract: Over the past years, deep learning on graphs has made significant progress in various areas, e.g., e-commerce, social networks, and healthcare. However, most existing graph learning tasks assume graphs are static, while real-world graphs may constantly grow or evolve. Therefore, it is crucial to study how to constantly adapt a graph learning model to new patterns/tasks over graphs without forgetting the previously learned knowledge. To this end, in this talk, I will introduce the newly emerging area of continual graph learning (CGL). Specifically, I will (1) introduce different continual graph learning settings and key challenges in the context of e-commerce/social networks, (2) present a general framework, i.e., Parameter Decoupled Graph Neural Networks (PDGNNs) with Topology-aware Embedding Memory (TEM), to perform continual learning over growing graphs, and (3) develop a Structural Knowledge Informed Continual Learning (SKI-CL) framework to perform multivariate time series forecasting under the continual learning setting, which leverages the structural knowledge to characterize the dynamic variable dependencies within each regime. I will also highlight my future research agenda at the end of the talk.
Bio: Dongjin Song is an assistant professor in the Department of Computer Science and Engineering at the University of Connecticut (UConn). His research interests include machine learning, deep learning, data mining, and related applications for time series data and graph representation learning. Papers describing his research have been published at top-tier data science and artificial intelligence conferences, such as NeurIPS, ICML, ICLR, KDD, ICDM, SDM, AAAI, IJCAI, CVPR, ICCV, etc. He has co-organized AI for Time Series (AI4TS) workshop at IJCAI 2022, 2023 and the Mining and Learning from Time Series workshop at KDD 2022, 2023. He has also served as senior PC for AAAI, IJCAI, and CIKM. He won the UConn Research Excellence Research (REP) Award in 2021. His research has been funded by NSF, USDA, Morgan Stanley, NEC Labs America, Travelers, etc.